Clasificación y mapeo automático de coberturas del suelo en imágenes satelitales utilizando Redes Neuronales Convolucionales
Classification and automatic mapping of land covers in satellite images using Convolutional Neural Networks
Contenido principal del artículo
Resumen
Descargas
Detalles del artículo
Palabras clave:
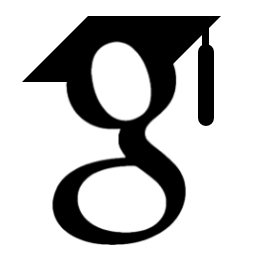
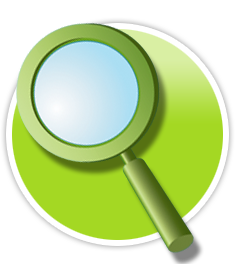
land cover
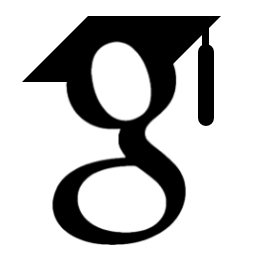
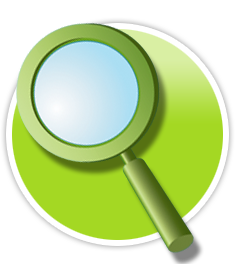
natural parks
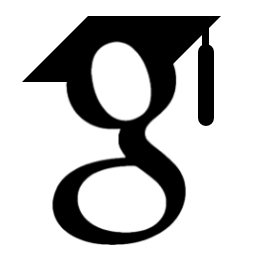
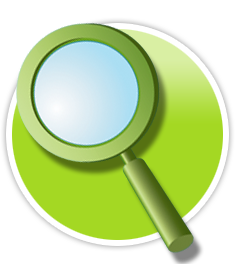
convolutional neural networks
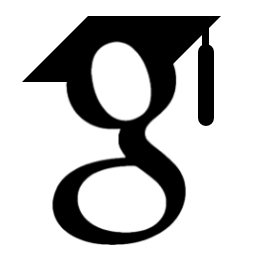
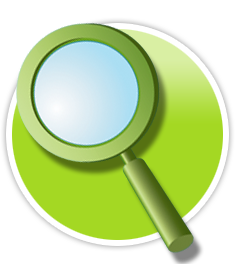
remote sensing
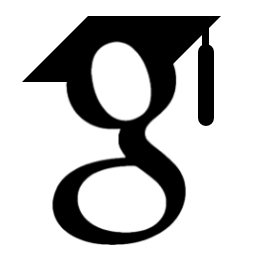
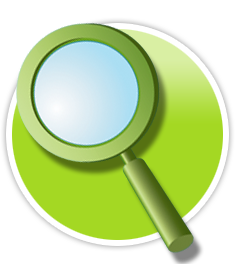
Aprendizagem de máquina
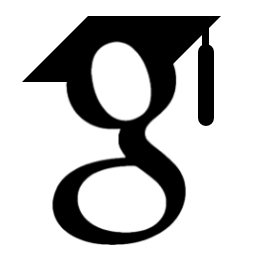
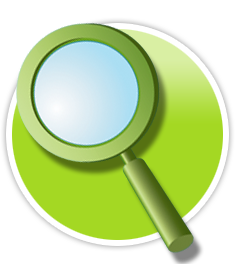
cobertura do solo
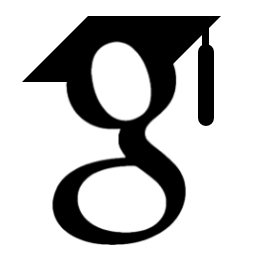
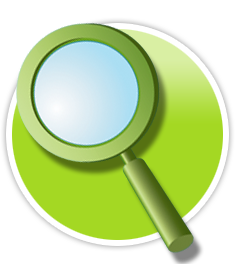
parques naturais
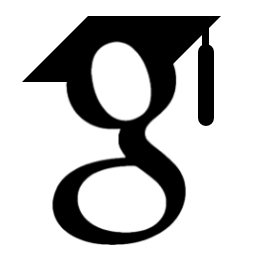
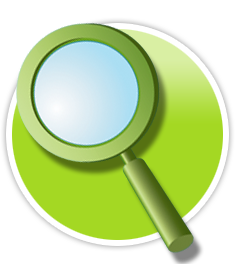
rede neural convolutional
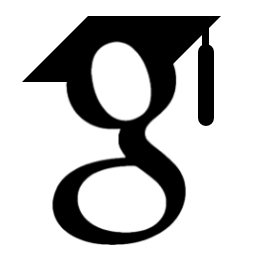
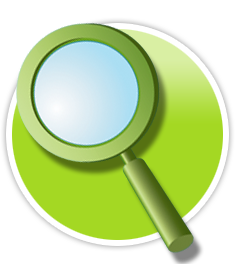
sensoriamento remoto
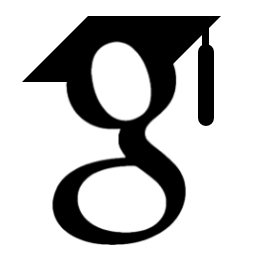
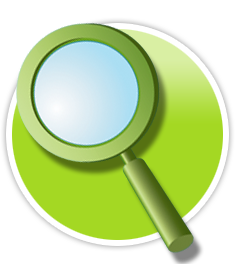
Aprendizaje automático
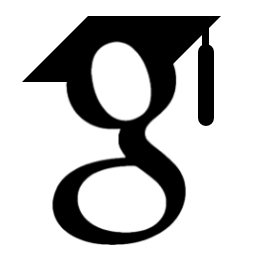
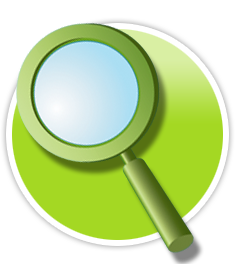
coberturas de suelo
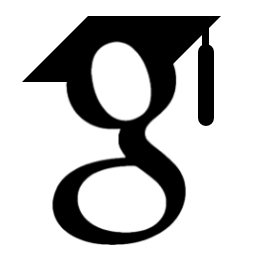
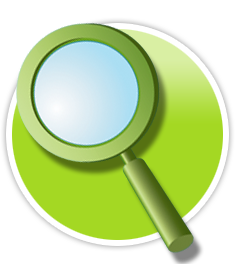
parques naturales
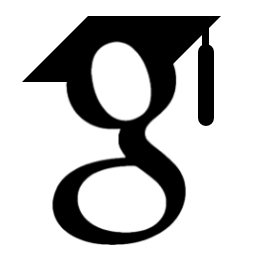
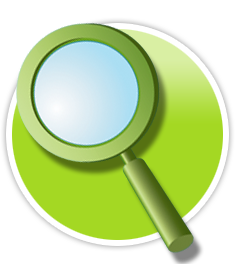
redes neuronales convolucionales
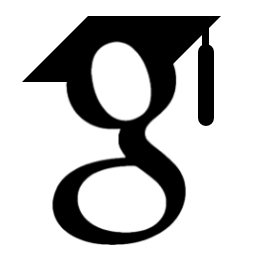
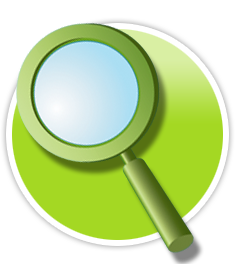
teledetección
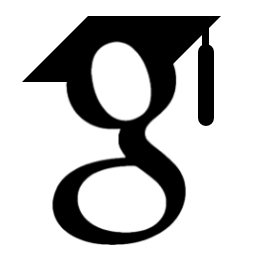
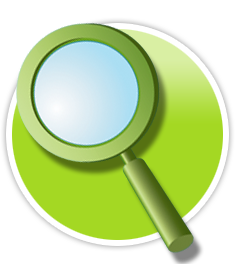
Referencias (VER)
Acevedo L, Grupo de planeacion y manejo, S. D. G. Y. M. D. A. P. (2012). Parques nacionales naturales de colombia. Retrieved from http://mapas.parquesnacionales.gov.co/#
Axesnet S.a.S. (2012). Sistema de Informacion Ambiental de Colombia - SIAC. Retrieved from http://www.siac.gov.co/Catalogo_mapas.html
Backoulou GF, Elliott NC, Giles KL, Mirik M. Processed multispectral imagery differentiates wheat crop stress caused bygreenbug from other causes. Computers and Electronics in Agriculture. 2015;115:34-39. https://doi.org/10.1016/j.compag.2015.05.008
Bokusheva R, Kogan F, Vitkovskaya I, Conradt S, Batyrbayeva M. Satellite-based vegetation health indices as a criteria for insuring against drought-related yield losses. Agricultural and Forest Meteorology. 2016;220:200-206. https://doi.org/10.1016/j.agrformet.2015.12.066
Cruz-Roa A, Arévalo J, Judkins A, Madabhushi A, González F. 2015. A method for medulloblastoma tumor differentiation based on convolutional neural networks and transfer learning. International Symposium on Medical Information Processing and Analysis, 9681, 968103. https://doi.org/10.1117/12.2208825
Cruz-Roa A, Basavanhally A, González F, Gilmore H, Feldman M, Ganesan S, Madabhushi A. Automatic detection of invasive ductal carcinoma in whole slide images with convolutional neural networks. Proc. SPIE, 2014;9041(216):904103-904115. https://doi.org/10.1117/12.2043872
Eisavi V, Homayouni S, Yazdi AM, Alimohammadi A. Land cover mapping based on random forest classification of multitemporal spectral and thermal images. Environmental Monitoring and Assessment. 2015;187(5):1-14. https://doi.org/10.1007/s10661-015-4489-3
Huang JT, Li J, Gong Y. 2015. An analysis of convolutional neural networks for speech recognition. In 2015 IEEE International Conference on Acoustics, Speech and Signal Processing (ICASSP) (pp. 4989–4993). IEEE. https://doi.org/10.1109/ICASSP.2015.7178920
IDEAM, IGAC, & CORMAGDALENA. (2008). Mapa de Cobertura de la Tierra Cuenca Magdalena-Cauca: Metodología CORINE Land Cover adaptada para Colombia a escala 1:100.000. Instituto de Hidrología, Meteorología y Estudios Ambientales, Instituto Geográfico Agustín Codazzi y Corporación Autónoma Regional del río Grande de la Magdalena (Vol. 1).
Krizhevsky A, Sutskever I, Hinton GE. 2012. ImageNet Classification with Deep Convolutional Neural Networks. Advances In Neural Information Processing Systems. 1–9. https://doi.org/http://dx.doi.org/10.1016/j.protcy.2014.09.007
Liu Y, Zhang B, Wang LM, Wang N. A self-trained semisupervised SVM approach to the remote sensing land cover classification. Computers and Geosciences. 2013;59:98-107. https://doi.org/10.1016/j.cageo.2013.03.024
Martin M, Newman S, Aber J, Congalton R. Determining forest species composition using high spectral resolution remote sensing data. Remote Sensing of Environment. 1998;65(3):249-254. https://doi.org/10.1016/S0034-4257(98)00035-2
Ministerio del Medio Ambiente. (2010). Leyenda nacional de coberturas de la tierra.
Perlin HA, Lopes HS. Extracting human attributes using a convolutional neural network approach. Pattern Recognition Letters. 2015;68:250-259. https://doi.org/10.1016/j.patrec.2015.07.012
Rodriguez-Galiano VF, Ghimire B, Rogan J, Chica-Olmo M, Rigol-Sanchez JP. An assessment of the effectiveness of a random forest classifier for land-cover classification. ISPRS Journal of Photogrammetry and Remote Sensing. 2012;(67):93-104. https://doi.org/10.1016/j.isprsjprs.2011.11.002
Rujoiu-Mare MR, Mihai B. Mapping Land Cover Using Remote Sensing Data and GIS Techniques: A Case Study of Prahova Subcarpathians. Procedia Environmental Sciences. 2016;32:244-255. https://doi.org/10.1016/j.proenv.2016.03.029
Thonfeld F, Feilhauer H, Braun M, Menz G. Robust Change Vector Analysis (RCVA) for multi-sensor very high resolution optical satellite data. International Journal of Applied Earth Observation and Geoinformation. 2016;50:131-140. https://doi.org/10.1016/j.jag.2016.03.009
Wang H, Cruz-Roa A, Basavanhally A, Gilmore H, Shih N, Feldman M, Madabhushi A. Mitosis detection in breast cancer pathology images by combining handcrafted and convolutional neural network features. Journal of Medical Imaging (Bellingham, Wash.). 2014;1(3):34003. https://doi.org/10.1117/1.JMI.1.3.034003
Warner TA, Foody GM, Nellis MD. 2009. The SAGE Handbook of Remote Sensing. 504. https://doi.org/10.4135/9780857021052
Zhang R, Zhu D. Study of land cover classification based on knowledge rules using high-resolution remote sensing images. Expert Systems with Applications, 2011;38(4):3647-3652. https://doi.org/10.1016/j.eswa.2010.09.019